The developing business era, and we are all in the race for top-notch technology. It is evident that the demands for more GPU computing power are greater than ever. Whether it’s the topic of applications in AI, machine learning, data science, or gaming, users get straight to their topic and take a call between on-premise GPU servers or cloud GPU solutions.
The inherent benefit of owning and running one’s own hardware is the high demand for GPUs due to their usability and advantages in high computing tasks. Thus, keeping in mind the relevant debate, on-premise GPUs vs. cloud GPU servers appear as the two dominant technologies that can take your website to new cloud hosting growth levels.
Whether it’s on-premise cloud or reliable GPU cloud servers, each option has its own strengths and limitations, suited for specific computational requirements, budgetary constraints, and long-term strategic goals of the individual or organization. This short guide will help you weigh the pros and cons of on-premise and cloud GPU solutions, guiding you to know which way is the best fit for your needs.
So let’s begin.
Table of Content
Understanding GPU Computing
The very first step in our blog will be clearing out the basics of what a cloud GPU server is.
In a simple description, we can say that a dedicated Graphics Processing Unit (GPU) is a processor designed to speed up graphics rendering processes. Today, they’ve come a long way as an incredibly powerful tool for executing complicated computations, especially high-parallel-processing operations.
Unlike the traditional ones, the Central Processing Unit (CPU), GPUs are optimized for executing hundreds, thousands, or even millions of smaller tasks in parallel.
A parallel task simulation means you can efficiently handle multiple tasks at the same time without lags and slow loading times. Therefore, GPUs are most effective for workloads like deep learning, image processing, and simulations.
Why GPU Computing Matters?
Advantages in GPU computing that make it important for high-performance computing:
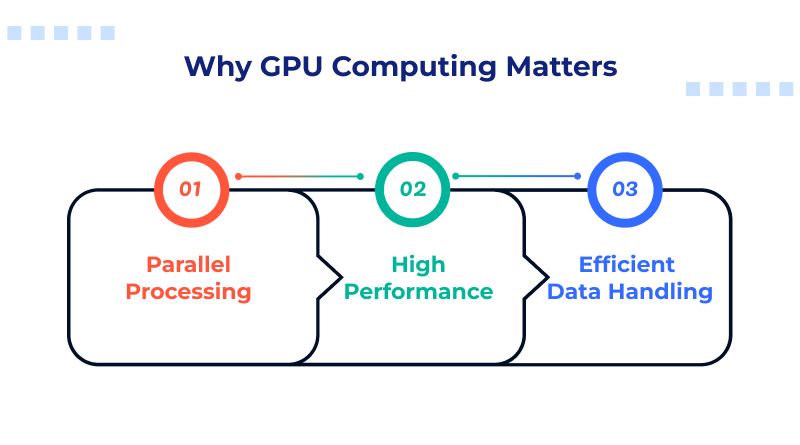
- Parallel Processing: Thousands of simultaneous computations handled by a GPU make data processing fast.
- High Performance: Through the acceleration of tasks, GPUs can minimize the time needed for complex computations significantly.
- Efficient Data Handling: In this regard, GPUs handle large datasets more efficiently than CPUs, providing faster results in applications that are data intensive.
Understanding On-Premise GPU
An on-premise GPU is simply the term used to refer to a graphics processing unit that is physically located in your data center or server room. This means you own the hardware and are responsible for its maintenance, power, and cooling.
Advantages of On-Premise GPU Solutions:
- Absolute Control: On-premise GPUs allow absolute control in terms of configurations, usage, and customization. The users tailor the hardware and software settings to specifically meet the task performed.
- Maximum Performance: The hardware can be configured into dedicated workloads that can minimize latency and effectively gain consistent high performance.
- Data Security: This solution generally offers advanced security for data because the sensitive data never leaves the local network. Hence, they tend to be preferred by industries that have stringent privacy regulations.
Disadvantages of On-Premise GPU Solutions:
- High Installation Cost: Installation of on-premise GPU infrastructure proves to be costly, as it requires a high upfront investment in hardware and infrastructure.
- Recurring Maintenance Cost: Because of the physical hardware, maintenance and upgrade processes occur along with incurring software updates, hardware repairs, and troubleshooting.
- Scalability Issues: On-premise configurations are usually quite challenging to scale. Introducing a resource incurs a wait for the complete procurement and integration of additional hardware and infrastructure.
Understanding Cloud GPU
A cloud GPU is a GPU server where all your data and operations are carried out through a remote cloud network. It can be accessed from any place over the internet.
It’s one of the services that reliable cloud providers like MilesWeb, AWS, GCP, Azure, and many others offer under their Cloud Computing Services. You’re essentially renting out the resources and paying as per their plans. Some offer pay-as-you-go, while others have customized plans with desired configurations.
Advantages of Cloud GPU Solutions:
- Pay-as-You-Go Model: With cloud service providers, there is always flexible pricing in place, so one will pay according to the usage. This is absolutely suited for temporary or fluctuating workloads.
- Speedy Deployment: Cloud GPUs can be configured and available in a matter of minutes. Thus, pilot projects would run much faster, and even testing could be conducted at a faster pace.
- Easy Management: The actual maintenance, updates, and hardware upgrades are taken care of by cloud providers like managed service providers, and this reduces technical support within one’s premises.
Disadvantages of Cloud GPU Solutions:
- Latency Issues: Accessing a GPU through a network comes with the potential issue of latency. Thus, the applications may then not be feasible in real-time.
- Vendor Lock-In: Many vendors have lock-in policies, which means users need to work within the ecosystem, which can be binding and expensive to switch out.
- Security Threats: While cloud computing is highly secured, many times it entails shared infrastructure, which poses a risk to highly sensitive data.
Consideration Factors for On-Premise vs. Cloud GPU Selection
When choosing between on-premise and cloud GPUs, several factors need consideration. So basically your choice should analyze the type for your workload, budget requirements, needs to scale up, and security.
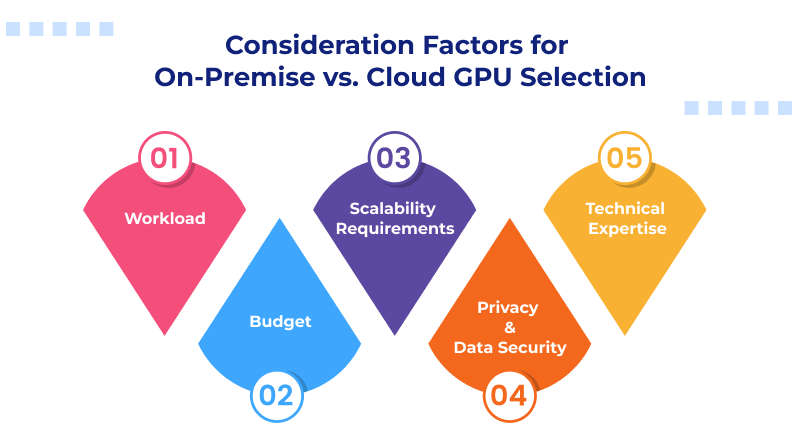
- Workload: Consider the specific computational needs of your application. For continuous, high-intensity workloads, on-premise GPUs seem to offer better performance; sporadic or variable workloads fit well.
- Budget: On-premises GPUs are pretty capital-intensive at the outset. Whereas you get more flexible, usage-based pricing with your GPU cloud server. Consider your available budget and patterns of usage.
- Scalability Requirements: If your workload scales high or varies, then it becomes much easier to handle them with cloud GPUs. On the other hand, on-premises solutions are a bit more costly when it comes to scaling them for high-performance and large-scale operations.
- Data Security and Privacy: In case the data you are dealing with is sensitive, then the stringent compliances of data-sensitive industries require control and security that can be achieved with on-premise GPUs.
- Technical Expertise: To keep the on-premise setups running, in-house expertise will always be needed. This includes everything from the basic steps like installation to complicated maintenance. Cloud options reduce that burden; however, technical knowledge is still good to have.
A Quick Overview Comparing On-Premise vs. Cloud GPU Servers
Criteria | On-Premise GPU Solutions | Cloud GPU Solutions |
Initial Investment | High capital costs in hardware and infrastructure. | Low up-front cost; pay as you go, no hardware costs to purchase. |
Maintenance | Annual maintenance must be steady due to equipment servicing and updates to computer software, requiring IT employees. | Maintenance is handed over to the cloud provider, so fewer in-house IT resources are required. |
Scalability | It is not flexible towards scalability. Scaling can only be done by buying other components of hardware that shall be integrated. | Scalability is extremely high; resources can be scaled up to meet demand. |
Performance Consistency | Predictable and consistent performance on customized workloads | Performance is dependent upon the network latency and shared resources on the cloud platform. |
Data Control & Security | Better control over data security and compliance. This is suitable for very sensitive data storage organizations and companies. | Shared infrastructure poses risks for sensitive data; compliance varies on policies developed by the service provider. |
Customization | High degree of personalization with customers as for the customization of hardware and software needs. | Less flexible with personalization; typically must run in the provider’s pre-set configurations. |
Geographical Flexibility | Locked to a fixed location, reliant on VPNs or other mechanisms to provide remote operation. | Accessible anywhere, anytime, with a network connection, and thus facilitates global collaboration and flexibility in working. |
Cost Over Time | It will be more expensive long-term for predictable workloads, as you only tend to purchase hardware once. | You can do cost optimization through managed cloud hosting services for short-term or varying uses. But it can be expensive in long-term, high-usage applications. |
Resource Utilization | Usage will be uncertain as users may leave some resources idle if the system is not optimized to perfection. | Resources can be scaled dynamically. That is, the right utilization can be achieved at present needs. |
Vendor Dependence | You will never be locked into a vendor. The owner owns 100% of the hardware and infrastructure. | It comes with a lock-in to a vendor; hence, it becomes tough to move data or applications to some other providers. |
Environmental Impact | You consume much more energy and require much more cooling, which results in a much larger carbon footprint. | Many cloud providers optimize for energy efficiency and use renewable energy sources. |
Access to Latest Hardware | You are bound by budget and cycles of upgrade, which makes it problematic to find newer models of the GPU. | Providers often provide access to the latest hardware without requiring an upgrade investment. |
AI and Machine Learning | Long-duration, high-compute workload with constant usage pattern. | Suitable for projects needing quick access to resources, such as model experimentation, or with lower budgets. |
Data Science | Good for continuous data analysis and handling large datasets securely. | Ideal for smaller-scale or variable-size projects; allows data scientists to spin up GPU instances as needed. |
Gaming and Virtual Reality | On-premises cloud GPU servers provide predictable, low-latency performance for real-time rendering and game development. | Leverages cloud GPU server scalability for cloud gaming but may incur latency, affecting real-time interaction quality. |
With the digital pace evolving daily, it is quite possible that shortly there will be AI accelerators and quantum computing, which may entirely change the face of GPU computing that we see today.
Hence, with the evolution of cloud technology for web development and other needs, the choice between on-premise vs. cloud GPU server solutions will likely include even more personalized options to address the needs of users at the point of need.
On-premise and cloud GPU solutions are uniquely advantageous in their ways. The on-premise GPUs are highly excellent for control, security, and performance for stable high-volume applications but have higher costs and maintenance.
On the contrary, cloud GPUs provide flexible, scalable, and cost-effective resources to be used in variable workloads with rapid project launches. The ideal selection between the two depends on your workload type, budget, scalability, and security needs.
FAQs
What are the benefits of on-premise GPUs?
On-premise GPUs offer full control over hardware. They can be deeply customized and optimized depending on the specific workload that is required—for example, high-performance AI training or real-time data processing. Generally, it has consistent and low-latency performance for processing speed. Data security improves since sensitive information stays within private infrastructure. In the case of stable workloads, on-premises cloud deployment also has upfront cost advantages over managed cloud services compared to where costs can be perpetual with cloud services.
What are the drawbacks of on-premise GPUs?
On-premise GPUs come with the very high costs associated with one-time purchases and installations of hardware as well as supporting infrastructure. Their maintenance and governance require dedicated IT resources and add costs in terms of staff, time, and equipment maintenance. Scaling such an on-premises solution is difficult because it requires further purchase of hardware plus space that may not always be available when you experience rapid growth or variations in demand.
How do I manage and maintain an on-premise GPU?
On-premises GPUs need continuous monitoring and checking, including the performance tuning aspect, firmware updates, and other driver installations, to reach optimal efficiency. Routine hardware maintenance is important, including checks on cooling systems, airflow, and even physical connections to avoid overheating or any form of hardware degradation. Most organizations track and monitor the patterns and possible issues resulting from the use of GPU monitoring software.
What are the costs to setup an on-premise GPU?
The setup of an on-premise GPU solution does not come for free. With the initial purchase of GPUs, prices can vary widely. It is probably going to cost thousands of dollars for every unit, depending on the specifications. Infrastructure needs in the form of server racks, cooling systems, and increased power supplies contribute to the cost of a setup on-premise. Installation and configuration are part of the expense, and many of these may require professional IT support or even specialists for successful system setup.
Long-term costs include the ongoing maintenance and likely upgrade costs of the equipment and electricity consumption, which can be costlier for some organizations to maintain on-premise solutions as compared to the pay-as-you-go option available through cloud services.
Is on-premise GPU a best fit for all AI/ML workloads?
An on-premise GPU server is the best for situations where low-latency processing is a priority and the cost of cloud resources exceeds an initial investment in hardware. However, workloads with variable demands or scaling requirements are not ideal for on-premise setups since there could be a need to fully exploit the flexibility offered by cloud GPUs. Therefore, for smaller projects or experiments that take less time, or even for some startups that are not in a position to invest much in infrastructure, cloud-based GPUs are much more flexible and cost-effective.